Talk: Building Human-AI Alignment, 4-5:30 Wed. May 1
Specifying, Inspecting, and Modeling AI Behaviors
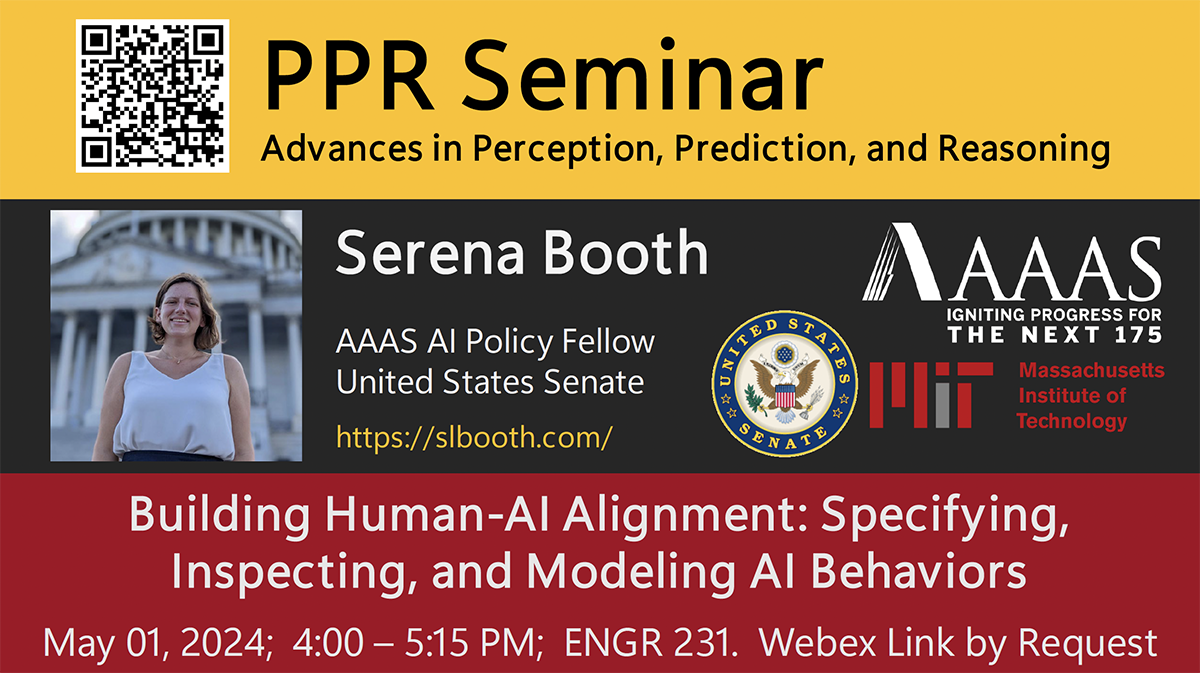
Building Human-AI Alignment: Specifying, Inspecting, and Modeling AI Behaviors
Serena Booth
AAAS AI Policy Fellow, United States Senate
AAAS AI Policy Fellow, United States Senate
4-5:30pm ET Wednesday, 1 May, 2024
UMBC ENGR 231, Webex Link by Request
The learned behaviors of AI and robot agents should align with the intentions of their human designers. Toward this goal, people must be able to easily specify, inspect, and model agent behaviors. For specifications, we will consider expert-written reward functions for reinforcement learning (RL) and non-expert preferences for reinforcement learning from human feedback (RLHF). I will show evidence that experts are bad at writing reward functions: even in a trivial setting, experts write specifications that are overfit to a particular RL algorithm, and they often write erroneous specifications for agents that fail to encode their true intent. Next, I will show that the common approach to learning a reward function from non-experts in RLHF uses an inductive bias that fails to encode how humans express preferences, and that our proposed bias better encodes human preferences both theoretically and empirically. For inspection, humans must be able to assess the behaviors an agent learns from a given specification. I will discuss a method to find settings that exhibit particular behaviors, like out-of-distribution failures. Lastly, cognitive science theories attempt to show how people build conceptual models that explain agent behaviors. I will show evidence that some of these theories are used in research to support humans, but that we can still build better curricula for modeling. Collectively, my research provides evidence that—even with the best of intentions— current human-AI systems often fail to induce alignment; my research proposes promising directions for how to build better aligned human-AI systems.
Serena Booth received her PhD at MIT CSAIL in 2023. Serena studies how people write specifications for AI systems and how people assess whether AI systems are successful in learning from specifications. While at MIT, Serena served as an inaugural Social and Ethical Responsible Computing Scholar, teaching AI Ethics and developing MIT’s AI ethics curriculum that is also released on MIT OpenCourseWare. Serena is a graduate of Harvard College (2016), after which she worked as an Associate Product Manager at Google to help scale Google’s ARCore augmented reality product to 100 million devices. Serena currently works in the U.S. Senate as a AAAS AI Policy Fellow, where she is working on AI policy questions for the Senate Banking, Housing, and Urban Affairs Committee. Her research has been supported by an MIT Presidential Fellowship and by an NSF GRFP. She is a Rising Star in EECS and an HRI Pioneer.
Tags:
Posted: April 30, 2024, 12:13 PM