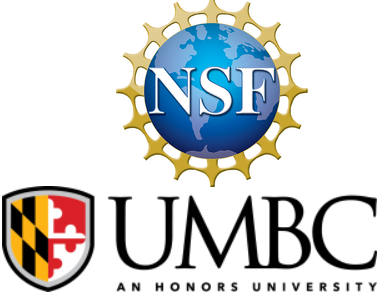
CAREER:
Synergistic Cross-IoT N-Way Sensing
Using Wireless Traffic in the Edge
NSF CNS-1652669
|
Passive-ZigBee: Enabling ZigBee Communication in IoT Networks with 1000X+ Less Power ConsumptionWithin heterogenous IoT sensor networks, users of ZigBee devices expect long-lasting battery usage due to its ultra-low power and duty cycle. In IoT networks, to demonstrate even further ultralow power consumption, we introduce Passive-ZigBee that demonstrates we can transform an existing productive WiFi signal into a ZigBee packet for a CoTS low-power consumption receiver while consuming 1,440 times lower power compared to traditional ZigBee. Moreover, this low power backscatter radio can bridge between the ZigBee and WiFi devices by relaying data allowing heterogenous radios to communicate with each other. We built a hardware prototype and implement these devices on a commodity ZigBee, WiFi, and an FPGA platform. Our experimental evaluation demonstrates the backscattered WiFi packets can be decoded by CoTS ZigBee receivers over a distance of 55 meters in none-line-of-sight and with human movements. Our Passive-ZigBee can consume only 25µW when transferring sensor data and relay ZigBee and WiFi data compared to traditional ZigBee (36mW). Our FPGA synthesis tool demonstrated the extremely low power consumption. This paper is awarded as Best Paper Runner-up Award and published at ACM SenSys 2018.[PDF] |
|
EAR: Exploiting Uncontrollable Ambient RF Signals in Heterogeneous Networks for Gesture RecognitionThe exponentially increasing number of Internet-of-Thing (IoT) devices introduces a spectrum crisis in the shared ISM band. However, it also introduces opportunities for conducting radio frequency (RF) sensing using pervasively available signals generated by heterogeneous IoT devices. In this paper, we explore how to leverage the ambient wireless traffic that i) generated by uncontrollable IoT devices and ii) sensed by ambient noise floor measurements (a widely available metric in IoT devices) for human gesture recognition. Specifically, we introduce our system EAR, which can conduct fine-grained human gesture recognition using coarse-grained measurements (i.e., noise floor) of ambient RF signals generated from uncontrollable signal sources. We conducted extensive evaluations in both residential and academic buildings. Experimental results show that although EAR uses coarse-grained noise floor measurements to sense the uncontrollable signal sources, the signal sources can be distinguished with an accuracy up to 99.76%. Moreover, EAR can recognize fine-grained human gestures with high accuracy even under extremely low traffic rate (i.e., 4%) from uncontrollable ambient signal sources. This paper is published at SenSys 2018.[PDF] |